Overview
Diverging from the classic random walk, the Node2Vec Walk is a biased random walk which can explore neighborhoods in a BFS as well as DFS fashion. Please refer to the Node2Vec algorithm for details.
Considerations
- Self-loops are also eligible to be traversed during the random walk.
- The Node2Vec Walk algorithm ignores the direction of edges but calculates them as undirected edges.
Example Graph
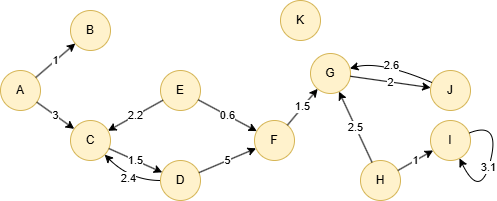
To create this graph:
// Runs each row separately in order in an empty graphset
create().edge_property(@default, "score", float)
insert().into(@default).nodes([{_id:"A"},{_id:"B"},{_id:"C"},{_id:"D"},{_id:"E"},{_id:"F"},{_id:"G"},{_id:"H"},{_id:"I"},{_id:"J"},{_id:"K"}])
insert().into(@default).edges([{_from:"A", _to:"B", score:1}, {_from:"A", _to:"C", score:3}, {_from:"C", _to:"D", score:1.5}, {_from:"D", _to:"C", score:2.4}, {_from:"D", _to:"F", score:5}, {_from:"E", _to:"C", score:2.2}, {_from:"E", _to:"F", score:0.6}, {_from:"F", _to:"G", score:1.5}, {_from:"G", _to:"J", score:2}, {_from:"H", _to:"G", score:2.5}, {_from:"H", _to:"I", score:1}, {_from:"I", _to:"I", score:3.1}, {_from:"J", _to:"G", score:2.6}])
Creating HDC Graph
To load the entire graph to the HDC server hdc-server-1
as hdc_node2vec_walk
:
CALL hdc.graph.create("hdc-server-1", "hdc_node2vec_walk", {
nodes: {"*": ["*"]},
edges: {"*": ["*"]},
direction: "undirected",
load_id: true,
update: "static",
query: "query",
default: false
})
hdc.graph.create("hdc_node2vec_walk", {
nodes: {"*": ["*"]},
edges: {"*": ["*"]},
direction: "undirected",
load_id: true,
update: "static",
query: "query",
default: false
}).to("hdc-server-1")
Parameters
Algorithm name: random_walk_node2vec
Name |
Type |
Spec |
Default |
Optional |
Description |
---|---|---|---|---|---|
ids |
[]_id |
/ | / | Yes | Specifies nodes to start random walk by their _id ; computes for all nodes if it is unset. |
uuids |
[]_uuid |
/ | / | Yes | Specifies nodes to start random walk by their _uuid ; computes for all nodes if it is unset. |
walk_length |
Integer | ≥1 | 1 |
Yes | Depth of each walk, i.e., the number of nodes to visit. |
walk_num |
Integer | ≥1 | 1 |
Yes | Number of walks to perform for each specified node. |
p |
Float | >0 | 1 |
Yes | The return parameter; a larger value reduces the probability of returning. |
q |
Float | >0 | 1 |
Yes | The in-out parameter; it tends to walk at the same level when the value is greater than 1, otherwise it tends to walk far away. |
edge_schema_property |
[]"<@schema.?><property> " |
/ | / | Yes | Numeric edge properties used as edge weights, summing values across the specified properties; edges without the specified properties are ignored. |
return_id_uuid |
String | uuid , id , both |
uuid |
Yes | Includes _uuid , _id , or both values to represent nodes in the results. |
limit |
Integer | ≥-1 | -1 |
Yes | Limits the number of results returned; -1 includes all results. |
File Writeback
CALL algo.random_walk_node2vec.write("hdc_node2vec_walk", {
params: {
return_id_uuid: "id",
walk_length: 6,
walk_num: 2,
p: 10000,
q: 0.0001
},
return_params: {
file: {
filename: "walks"
}
}
})
algo(random_walk_node2vec).params({
projection: "hdc_node2vec_walk",
return_id_uuid: "id",
walk_length: 6,
walk_num: 2,
p: 10000,
q: 0.0001
}).write({
file:{
filename: 'walks'
}})
Result:
_ids
J,G,F,D,C,E,
D,C,A,B,A,C,
F,G,E,C,A,B,
H,I,I,H,G,F,
B,A,C,D,F,G,
A,B,A,B,A,C,
E,C,E,C,A,B,
K,
C,E,F,G,J,G,
I,I,H,G,F,E,
G,H,I,I,H,G,
J,G,F,D,C,E,
D,C,A,B,A,C,
F,E,C,D,F,E,
H,G,H,G,J,G,
B,A,C,D,F,G,
A,C,D,F,E,C,
E,C,E,C,A,B,
K,
C,A,B,A,C,D,
I,H,G,J,G,H,
G,H,I,I,H,G,
Full Return
CALL algo.random_walk_node2vec("hdc_node2vec_walk", {
params: {
return_id_uuid: "id",
ids: ['J'],
walk_length: 6,
walk_num: 3,
p: 2000,
q: 0.001
},
return_params: {}
}) YIELD walks
RETURN walks
exec{
algo(random_walk_node2vec).params({
return_id_uuid: "id",
ids: ['J'],
walk_length: 6,
walk_num: 3,
p: 2000,
q: 0.001
}) as walks
return walks
} on hdc_node2vec_walk
Result:
_ids |
---|
["J","G","F","D","C","E"] |
["J","G","J","G","F","D"] |
["J","G","J","G","H","I"] |
Stream Return
CALL algo.random_walk_node2vec("hdc_node2vec_walk", {
params: {
return_id_uuid: "id",
ids: ['A'],
walk_length: 5,
walk_num: 10,
p: 1000,
q: 1,
edge_schema_property: 'score'
},
return_params: {
stream: {}
}
}) YIELD walks
RETURN walks
exec{
algo(random_walk_node2vec).params({
return_id_uuid: "id",
ids: ['A'],
walk_length: 5,
walk_num: 10,
p: 1000,
q: 1,
edge_schema_property: 'score'
}).stream() as walks
return walks
} on hdc_node2vec_walk
Result:
_ids |
---|
["A","C","A","D","C"] |
["A","C","A","C","A"] |
["A","C","A","D","A"] |
["A","C","A","C","A"] |
["A","C","A","D","E"] |
["A","C","A","D","E"] |
["A","C","A","B","A"] |
["A","C","A","D","A"] |
["A","C","A","C","D"] |
["A","C","A","C","A"] |