Overview
Betweenness centrality quantifies the likelihood that a node lies on the shortest paths between any two other nodes. This metric effectively identifies "bridge" nodes that facilitate connectivity between different parts of a graph.
Betweenness centrality values range from 0 to 1, with higher scores indicating nodes that exert greater influence over the flow and connectivity of the network.
References:
- L.C. Freeman, A Set of Measures of Centrality Based on Betweenness (1977)
- L.C. Freeman, Centrality in Social Networks Conceptual Clarification (1978)
Concepts
Shortest Path
The shortest paths between two nodes are the paths that contain the fewest edges. When considering edge weights, the (weighted) shortest paths are those with the lowest total weight sum.
Betweenness Centrality
Betweenness centrality of a node x
is computed by:
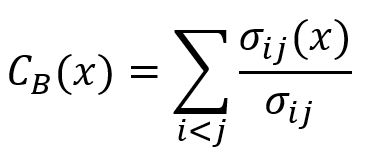
where,
i
andj
are two distinct nodes in the graph, excludingx
.σij
is the total number of shortest paths betweeni
andj
.σij(x)
is the number of shortest paths betweeni
andj
that pass through nodex
.σij(x)/σij
gives the probability thatx
lies in the shortest paths betweeni
andj
. Note that ifi
andj
are not connected,σij(x)/σij
is 0.
The final value is normalized by the factor (k – 1)(k – 2)/2
, where k
is the total number of nodes in the graph. This normalization ensures the result lies within a fixed range, making it comparable across graphs of different sizes.
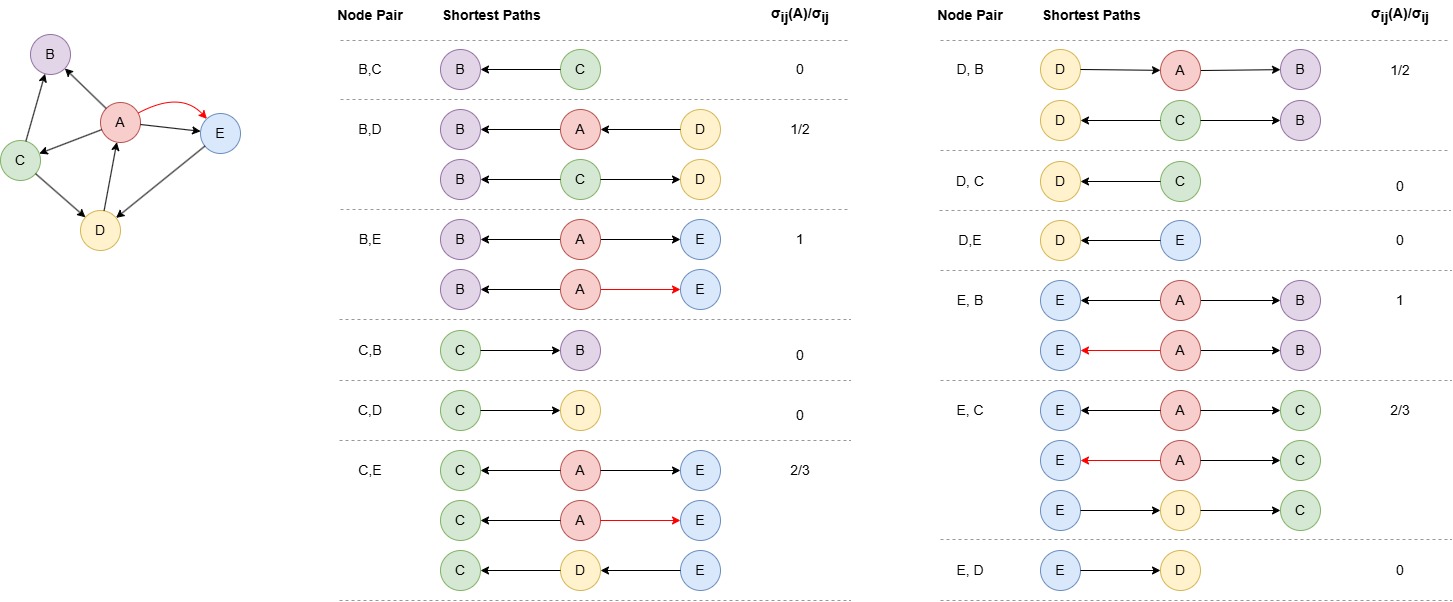
The betweenness centrality of node A
is computed as: (1/2 + 1 + 2/3 + 1/2 + 1 + 2/3) / (4 * 3 / 2) = 0.722222
.
Sampling
This algorithm requires substantial computational resources when applied to large graphs. When the number of nodes in a graph exceeds 10,000, it is recommended to sample by nodes or edges for an approximate computation. The algorithm performs a single uniform sampling.
Example Graph
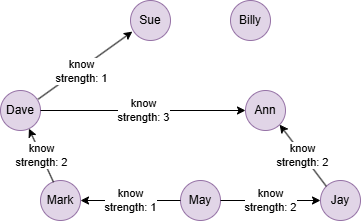
To create this graph:
// Runs each row separately in order in an empty graphset
create().node_schema("user").edge_schema("know")
create().edge_property(@know, "strength", int32)
insert().into(@user).nodes([{_id:"Sue"}, {_id:"Dave"}, {_id:"Ann"}, {_id:"Mark"}, {_id:"May"}, {_id:"Jay"}, {_id:"Billy"}])
insert().into(@know).edges([{_from:"Dave", _to:"Sue", strength:1}, {_from:"Dave", _to:"Ann", strength:3}, {_from:"Mark", _to:"Dave", strength:2}, {_from:"May", _to:"Mark", strength:1}, {_from:"May", _to:"Jay", strength:2}, {_from:"Jay", _to:"Ann", strength:2}])
Running on HDC Graphs
Creating HDC Graph
To load the entire graph to the HDC server hdc-server-1
as hdc_betweenness
:
CALL hdc.graph.create("hdc-server-1", "hdc_betweenness", {
nodes: {"*": ["*"]},
edges: {"*": ["*"]},
direction: "undirected",
load_id: true,
update: "static",
query: "query",
default: false
})
hdc.graph.create("hdc_betweenness", {
nodes: {"*": ["*"]},
edges: {"*": ["*"]},
direction: "undirected",
load_id: true,
update: "static",
query: "query",
default: false
}).to("hdc-server-1")
Parameters
Algorithm name: betweenness_centrality
Name |
Type |
Spec |
Default |
Optional |
Description |
---|---|---|---|---|---|
edge_schema_property |
[]"<@schema.?><property> " |
/ | / | Yes | Numeric edge properties used as weights, summing values across the specified properties; edges without this property are ignored. |
impl_type |
String | dijkstra , spfa |
dijkstra |
Yes | Specifies the weighted shortest paths to be computed by the Dijkstra or SPFA shortest path algorithm. This is only valid when edge_schema_property is used. |
sample_size |
Integer | -1 , -2 , [1, |V|] |
-2 |
Yes | Sets to -1 to sample log10(|V|) nodes (|V| is total number of nodes in the graph), or sets a custom number between [1, |V|] ; sets to -2 to perform no sampling. |
max_path_length |
Integer | >0 | / | Yes | Limits the shortest paths considered to those with a length no greater than this value. Note that this doesn't affect the total number of node pairs evaluated. |
return_id_uuid |
String | uuid , id , both |
uuid |
Yes | Includes _uuid , _id , or both to represent nodes in the results. |
limit |
Integer | ≥-1 | -1 |
Yes | Limits the number of results returned; -1 includes all results. |
order |
String | asc , desc |
/ | Yes | Sorts the results by betweenness_centrality . |
File Writeback
CALL algo.betweenness_centrality.write("hdc_betweenness", {
params: {
return_id_uuid: "id"
},
return_params: {
file: {
filename: "betweenness_centrality"
}
}
})
algo(betweenness_centrality).params({
projection: "hdc_betweenness",
return_id_uuid: "id"
}).write({
file: {
filename: "betweenness_centrality"
}
})
Result:
_id,betweenness_centrality
Dave,0.666667
Billy,0
May,0.133333
Mark,0.266667
Jay,0.133333
Ann,0.266667
Sue,0
DB Writeback
Writes the betweenness_centrality
values from the results to the specified node property. The property type is float
.
CALL algo.betweenness_centrality.write("hdc_betweenness", {
params: {},
return_params: {
db: {
property: 'bc'
}
}
})
algo(betweenness_centrality).params({
projection: "hdc_betweenness"
}).write({
db:{
property: 'bc'
}
})
Full Return
CALL algo.betweenness_centrality("hdc_betweenness", {
params: {
max_path_length: 2,
return_id_uuid: "id",
order: "desc",
limit: 3
},
return_params: {}
}) YIELD bc
RETURN bc
exec{
algo(betweenness_centrality).params({
max_path_length: 2,
return_id_uuid: "id",
order: "desc",
limit: 3
}) as bc
return bc
} on hdc_betweenness
Result:
_id | betweenness_centrality |
---|---|
Dave | 0.4 |
May | 0.133333 |
Mark | 0.133333 |
Stream Return
CALL algo.betweenness_centrality("hdc_betweenness", {
params: {
return_id_uuid: "id",
edge_schema_property: "strength"
},
return_params: {
stream: {}
}
}) YIELD r
FILTER r.betweenness_centrality > 0.6
RETURN r
exec{
algo(betweenness_centrality).params({
return_id_uuid: "id",
edge_schema_property: "strength"
}).stream() as r
where r.betweenness_centrality > 0.6
return r
} on hdc_betweenness
Result:
_id | betweenness_centrality |
---|---|
Dave | 0.6 |
Running on Distributed Projections
Creating Distributed Projection
To project the entire graph to its shard servers as dist_betweenness
:
create().projection("dist_betweenness", {
nodes: {"*": ["*"]},
edges: {"*": ["*"]},
direction: "undirected",
load_id: true
})
Parameters
Algorithm name: betweenness_centrality
Name |
Type |
Spec |
Default |
Optional |
Description |
---|---|---|---|---|---|
sample_rate |
Float | (0, 1] | 1 | Yes | Specifies the proportion of edges to sample for computation. |
limit |
Integer | ≥-1 | -1 |
Yes | Limits the number of results returned; -1 includes all results. |
order |
String | asc , desc |
/ | Yes | Sorts the results by betweenness_centrality . |
File Writeback
CALL algo.betweenness_centrality.write("dist_betweenness", {
params: {},
return_params: {
file: {
filename: "betweenness_centrality"
}
}
})
algo(betweenness_centrality).params({
projection: "dist_betweenness"
}).write({
file: {
filename: "betweenness_centrality"
}
})
Result:
_id,betweenness_centrality
Mark,0.133333
Jay,0.0666667
Ann,0.133333
Sue,0
Dave,0.333333
Billy,0
May,0.0666667
DB Writeback
Writes the betweenness_centrality
values from the results to the specified node property. The property type is double
.
CALL algo.betweenness_centrality.write("dist_betweenness", {
params: {},
return_params: {
db: {
property: 'bc'
}
}
})
algo(betweenness_centrality).params({
projection: "dist_betweenness"
}).write({
db:{
property: 'bc'
}
})