High-Density Parallel Graph Computing (shortened as HDPC hereafter) is a term fully coined by the R&D team at Ultipa. It essentially is a patent-pending technology that fully exposes the underpinning CPU hardware's parallel-execution capabilities.
Before Ultipa, no other graph databases or graph computing systems have pushed the limit of hardware's parallel capabilities. Taking Neo4j as an example, its typical enterprise-level deployment uses only three 8-core-CPU instances. There is ONLY 1 instance online performing while the other two are hot standbys. The maximum concurrency of the system is 4 threads, that is to say, each graph query has a maximum concurrency of 400%. Another example is graph computing using Python NetworkX, which only utilizes 1 thread of the CPU, and is considered a complete waste of computing power.
On the contrary, the concurrency capability is linearly scalable with the underlying hardware in the Ultipa Graph System, that is, a 32-vCPU system yields a concurrency of 3200%, and 6400% for that of a 64-vCPU, so on and so forth. As the numbers of instances grow in an Ultipa cluster, the computing power grows almost linearly.
There are two instant benefits of this linear scalability and high-density parallel computing:
- Exponential increment of the overall system performance and throughput, think 6400% vs 400% is 16-fold;
- The improvement of resource utilization: gives full play to the parallel ability of the modern CPU. Lower TCO, lower carbon emission.
The parallel computing of CPU relies on the underlying concurrent data structure, which makes possible the capability of deep graph traversal and dynamic pruning. The combination of these two capabilities empowers many business scenarios. For example, liquidity risk management, attribution analysis, performance analysis, in-depth correlation analysis, K-Hop influence evaluation, etc., and are all basic functions widely used in scenarios such as risk management, treasury management, anti-fraud, precision marketing, supply chain network, etc.
Many graph operations, such as whole-graph analysis, rely on graph algorithms, of which a typical feature is to traverse the whole graph reiteratively, which requires a huge amount of computing power. However, those graph algorithms published by the academic communities are mostly done in a sequential manner (such as the Louvain community detection algorithm) and are too slow and too impractical to serve in the commercial environment if not being modified to leverage parallel methodology such as HDPC.
A comparison shows the difference: it takes 10 hours to complete Louvain community detection with Python NetworkX on a graph dataset with only 1 million nodes and edges, and 3 hours with Huawei's GES graph system, while Ultipa has it done instantly -- literally in less than 0.1 seconds (<100 milliseconds). This performance gap is of tens of thousands of times.
In a recent benchmark test using the popular Twitter (2010) dataset, Ultipa shows a great performance advantage over other graph database systems (Neo4j, Tigergraph, and ArangoDB).
Here is a recap:
- Dataset: Twitter(2010), 41.6M Vertices and 1470M Edges
- It is imperative to point out that, Ultipa consistently performs consistently on any dataset. Twitter is picked only for ease-of-comparison.
- Testing Bed: 3-instance cluster over public cloud, each configured with Intel Xeon 2GHz, 32-vCPU CPU, 256GB DRAM, 1TB HDD (cloud-disk).
- Performance Testing on:
- Data Loading: Ultipa is 2-to-15x faster than most of graph databases, except for Tigergraph which loads similar to Ultipa .
- K-Hop: Ultipa is 10-1000x faster. For ultra-deep queries, Ultipa is the only system that can return with results, all other systems crash or never return.
- All Shortest Paths: Ultipa is 50x faster than TigerGraph.
- Graph Algorithms (PageRank, LPA, Louvain, Jaccard Similarity, etc.): Ultipa is at least 10x faster than Tiger.
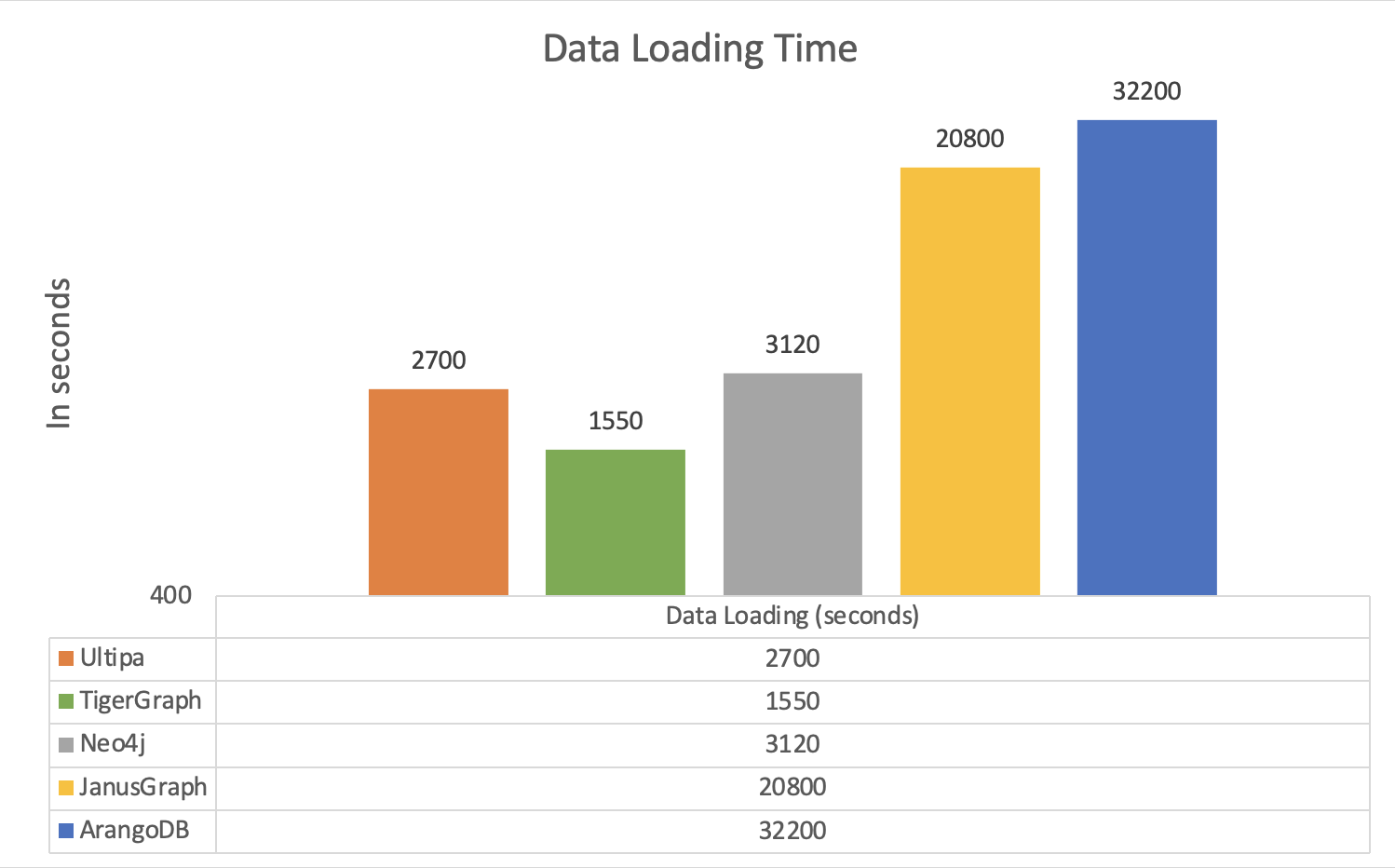
.png)
.png)
.png)
Click here to access the full report.